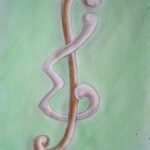
In the context of today’s big data and information age, blended learning has gradually entered people’s vision. At present, the number of business Japanese learners in China is increasing, and the breadth and depth of business Japanese education are expanding in all aspects. Therefore, people gradually pay attention to the practice and exploration mode of Internet, Japanese teaching and mixed learning, and learning evaluation is an important part of them. In this study, the construction of hybrid learning evaluation index is studied in the form of algorithm optimization and experimental verification. It takes blended learning as the evaluation object, aiming to build an evaluation index system of blended learning and help teachers implement high-quality blended learning. Based on the learning theory, this study also proposes the guiding strategies for senior high school students’ business Japanese learning from the perspective of schools, teachers and students themselves. Through empirical research and hypothesis verification, it provides reference for business Japanese education and learning in senior high schools in China. The experimental results show that the Nivre model after our calculation and optimization is based on the new education concept and the era development environment, and constantly improves the mixed learning mode, which improves the quality of business Japanese learning. Through correlation analysis and reliability and validity tests, the accuracy of the model in different test sets exceeded 92\%, which was in line with expected assumptions and empirical tests. The model has certain practical value.
With development of Internet of Things, big data and artificial intelligence, cell phone signaling data, point-of-interest data and machine learning methods have been widely used in research of various fields of transportation. The use of big data processing techniques and machine learning methods to mine intercity travel data collected by various types of traffic detectors provides a new way of thinking to study travel mode selection behavior. In this paper, we pre-processed cell phone signaling data, geospatial data and interest point data around three aspects: personal attributes, travel attributes and travel mode attributes, and designed intercity travel target group extraction, travel chain extraction, travel mode extraction and travel purpose extraction algorithms, which provide basis for travel feature analysis and travel mode choice behavior prediction modeling.
1970-2025 CP (Manitoba, Canada) unless otherwise stated.