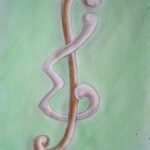
The combination of deep learning and digital media technology provides great scope for content creation. The article uses Generative Adversarial Network (GAN) in deep learning for content generation. Based on the three major forms of digital media content (image, audio, and video), image, audio, and video are generated by U-Net_GAN model, MAS-GAN model, and SSFLVGAN model, respectively, to construct a digital media content generation model based on generative adversarial networks. Subsequently, the model is validated for performance and the generated images, audio and video are evaluated for effectiveness. By studying the shortcomings of digital media content generation, we propose suggestions to improve its dissemination effect. The U-Net_GAN model outperforms other image generation models in all the indexes of generating images. The performance of speech generation and enhancement of MAS-GAN is much better than other audio generation and enhancement models. The average score of HDR video generated by SSFLVGAN is 4.20, and the average DMOS score is 5.97. The average DMOS score of SSFLVGAN is 5.97. DMOS score is 5.97, which are both 0.16 points higher than the traditional scheme. SSFLVGAN and the traditional scheme are comparable in terms of the picture impact of the generated video. The picture detail effect of the SSFLVGAN generated video is much better than the traditional scheme.
Based on the realization path of new quality productivity on industrial transformation and upgrading as well as talent supply, this paper carries out theoretical analysis in three directions, namely, direct effect, indirect effect and non-linear characteristics, and puts forward relevant research hypotheses. The panel data benchmark regression model, mediation effect model and threshold regression model are constructed respectively to verify the proposed hypotheses. The panel entropy method is used to measure the level of new quality productivity and carry out related research on the driving of new quality productivity. The partial differential decomposition of the spatial Durbin model shows that the direct and indirect effects of the new quality productivity are significantly positive at the 5% and 1% levels, respectively, indicating that the new quality productivity can promote the upgrading of industrial structure in the theoretical provinces. Introducing the variable of technological innovation for the mediation effect test, by observing columns (1) and (2), the regression coefficients of new quality productivity on technological innovation and new quality productivity on industrial structure upgrading are both positive at 0.2484 and 0.2048, respectively, which indicates that regional technology plays a mediating effect in the influence of new quality productivity on industrial structure upgrading.
Aiming at the inadequacy of the existing prediction models for time series data based on variables, this paper proposes an LSTM model based on fused multi-scale convolutional attention (MCA-LSTM). The experimental parameters are designed, the stock price dataset is constructed, and the modeling and prediction of the improved LSTM model is carried out using the closing price of individual stocks as the data base. And the model prediction accuracy was evaluated using RMSE, MAPE, and MAD indicators as evaluation indicators. In order to test the performance of MVA-LSTM portfolio model in arbitrage and generalization, the indicator factors are set to compare and analyze the application performance of MCA-LSTM-BL model. Using the framework of the mean semi-absolute deviation (MSAD) portfolio optimization model, a new portfolio optimization model based on return forecasting is developed. That is, the portfolio optimization model based on improved LSTM network return prediction (MCA-LSTM+MSAD). The asset values of different portfolio models are compared and the return prediction of each portfolio model is analyzed under the consideration of transaction costs. The MCA-LSTM+MSAD portfolio optimization model proposed in this paper is able to achieve an excess return of 56.98%. The MCA-LSTM+MASD model is able to maintain the maximum value consistently during the stock trading days of the study sample. This paper concludes that MCA-LSTM+MASD is a portfolio optimization model that can be further deepened and applied in real investment.
With the wide application of deep learning in the field of education, student emotion perception has become one of the research hotspots. The study recognizes learners’ facial expressions by face detection algorithm after collecting learners’ data and preprocessing. Algorithms such as convolutional neural network and ConvLSTM are used to recognize learners’ emotions, and learners’ emotions are constructed to be modeled. Evaluate the learner emotion performance of this paper’s model and compare it with other emotion recognition models. The model of this paper is used for practical research to collect students’ emotions in six classes, and statistics and analysis are performed. Finally, by studying the relationship between students’ emotions and behaviors, targeted suggestions for improving students’ behaviors are proposed. The accuracy of this paper’s model in recognizing student emotions on the RAF-DB dataset and classroom dataset is 90.32% and 97.65%, respectively, which is much higher than that of other pre-trained models. The recognition accuracy of this paper’s model for eight types of student emotions is between [0.93, 0.98]. In the statistics of classroom students’ emotions, the main emotions of students in session 1 were concentration, in session 2 were surprise and concentration, in sessions 3, 4, and 6 were surprise and delight, and in session 5 were concentration and disappointment. Focus was significantly positively correlated with “serious attendance”, “thinking”, “answering questions”, “discussing” and “doing tests”, tiredness was significantly positively correlated with “answering questions”, “reviewing” and “deserting”, boredom was significantly positively correlated with “answering questions”, “doing quizzes”, “reviewing” and “desertion”, doubts were significantly positively correlated with “discussing”, “doing quizzes” and “reviewing”, distraction was significantly positively correlated with “reviewing” and “desertion”, happiness was significantly positively correlated with “discussion”, and disappointment was significantly positively correlated with “desertion”.
Under the background of mediatized society, the fusion of reality and reality between the real world and cyberspace has made the role of social network public opinion more and more significant, and the occurrence of any major emergencies will trigger network public opinion. In this paper, the TF-IDF algorithm is used to extract the feature items of social media opinion data, synthesize them into text vectors and input them into the LDA topic model to mine the opinion topic words, and then combine the co-occurrence of the key topic words to draw the semantic maps of the opinion topic words on the web, so as to explore the dynamic evolution of the opinion topic words. The opinion text vectors are then used as inputs to extract the local features of the opinion text through CNN model, combine with BiLSTM model to obtain the global features and temporal information of the opinion text, and realize the dynamic prediction of opinion sentiment through SoftMax classifier. Taking the Xin Guan epidemic event as an example, and divided into three phases: latent period, outbreak period and recession period, the number of public opinion comments on microblog platform during the outbreak period can reach 1942.59 comments/day, and the evolution of public opinion topic words in different public opinion phases are dominated by themes such as “epidemic”, “pneumonia” and so on. When the CNN-BiLSTM model is used to predict the public opinion sentiment dynamics, the prediction accuracy is between 95.84% and 97.56%. Through the effective use of deep learning technology, it can clarify the orientation of public opinion development driven by social media data and provide reliable data support for social media public opinion guidance.
1970-2025 CP (Manitoba, Canada) unless otherwise stated.