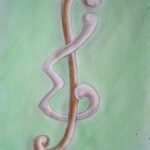
The lens array is a multi-functional optical element, which can modulate the incident light such as diffusion, beam shaping, light splitting, and optical focusing, thereby achieving large viewing angle, low aberration, small distortion, high temporal resolution, and infinite depth of field. Meanwhile, it has important application potential in the form, intelligence and integration of op-to electronic devices and optical systems. In this paper, the optical principle and development history of lens arrays are introduced, and the lens array fabrication technologies such as ink jet printing, laser direct writing, screen printing, photo lithography, photo polymerization, hot melt reflow and chemical vapor deposition are reviewed. The application progress of lens arrays in imaging sensing, illumination light source, display and photovoltaic fields is presented. And this paper prospected the development direction of lens arrays, and discussed the development trends and future challenges of new directions such as curved lenses, superimposed compound eye systems, and the combination of lenses and new op-to electronic materials.
Innovation and entrepreneurship and education have become an important topic in China’s higher education. Based on pedagogy theory, this paper divides innovation and entrepreneurship education in universities into three levels: ideological education, innovative education and entrepreneurial education. Innovation is the content of higher education, and it is also the ability that contemporary college students must have. Only with education can there be innovation, only with innovation can there be entrepreneurship, and only with entrepreneurship can there be innovation. This is of great significance to the development of multi-level education, universal education, innovation and entrepreneurship education, and the improvement of education, teaching and child-rearing levels. In order to promote the optimization practice of college students’ innovation and entrepreneurship education, this paper designs a software system which is convenient for college students’ project application, project implementation, data verification and progress report. At the same time, it can help people review and select team members, thus greatly improving management efficiency.
People’s aesthetic requirements for landscape environment are improving, and we can also see very beautiful as well as characteristic urban parks, street side green areas and scenic spots with certain aesthetic value around us, and we can find that people’s demand for the living environment they live in regarding beauty is also strengthening. The synergistic development of edge computing and cloud computing is an important development trend in the future, and integrating them into landscape design is an inevitable choice and requirement for developing gardens and building a beautiful China. Based on this, this study first proposes a methodological framework based on machine learning to model and predict GSS, and then proposes a data-driven multi-style terrain synthesis method. The experimental results prove that the optimized landscape perception model optimizes the landscape path aesthetics according to the relevant theories and actual cases of landscape planning and construction.
Multimode fibre optic communication systems, employing mode/mode group multiplexing, present challenges in accurately identifying numerous modes and mode groups for improved performance. In this study, we propose an intelligent identification model utilizing a fully convolutional neural network (CNN) to precisely identify multimode fibre modes and their clusters. The model is simulated and experimentally validated, considering noise influences on linear polarisation modes. Using a platform with OM2 multimode fibre and a multiplane optical conversion mode multiplexer, we capture optical field information for 10 modes and their corresponding mode groups. Extensive data are employed for training and validation, achieving a 100% recognition rate for all modes and mode groups in experiments. Notably, when employing a 44-photodetector array, an impressive 98.3% recognition efficiency is attained, showcasing the potential of deep learning in advancing multimode fibre optic communication systems.
To address the human activity recognition problem and its application in practical situations, a CNN-LSTM hybrid neural network model capable of automatically extracting sensor data features and memorizing temporal activity data is designed and improved by integrating CNN and gated recurrent units as a variant of RNN. A multi-channel spatiotemporal fusion network-based two-person interaction behavior recognition method is proposed for two-person skeletal sequential behavior recognition. Firstly, a viewpoint invariant feature extraction method is used to extract two-player skeleton features, then a two-layer cascaded spatiotemporal fusion network model is designed, and finally, a multi-channel spatiotemporal fusion network is used to learn multiple sets of two-player skeleton features separately to obtain multi-channel fusion features, and the fusion features are used to recognize the interaction behavior, and the weights are shared among the channels. Applying the algorithm in the paper to the UCF101 dataset for experiments, the accuracy of the two-person cross-object experiment can reach 96.42% and the accuracy of the cross-view experiment can reach 97.46%. The method in the paper shows better performance in two-player interaction behavior recognition compared to typical methods in this field.
Taekwondo behavior recognition has become a popular study issue in the past few decades due to its vast range of applications in the visual realm. The research of Taekwondo behavior recognition based on skeleton sequences has received increasing attention in recent years due to the widespread use of depth sensors and the development of real-time skeleton estimate methods based on depth images. In order to characterize the behavioral sequences, the majority of research work currently in existence extracts the spatial domain information of various skeleton joints within frames and the temporal domain information of the skeleton joints between frames. However, this research work ignores the fact that different joints and postures play different roles in determining the behavioral categories. Consequently, this paper presents a spatio-temporal weighted gesture Taekwondo features-based approach for Taekwondo recognition that employs a bilinear classifier to iteratively compute the weights of the static gestures and joint points relative to the action category in order to identify the joint points and gestures with high information content; concurrently, this paper introduces dynamic temporal regularization and Fourier time pyramid algorithms for temporal modeling in order to provide a better temporal analysis of the behavioural features, and ultimately employs support vector machines to complete the behavioural classification. According to experimental results on several datasets, this strategy outperforms certain other methods in terms of recognition accuracy and is highly competitive.
A large amount of course data has been accumulated in the long-term teaching activities of universities. It is of great research value to use the data resources to analyze the course teaching status and provide decision support for improving the course teaching quality. In this paper, we design and implement a course evaluation system based on association rules and cluster analysis, analyze the functional requirements of the course evaluation system, and pre-process the course evaluation data. Students’ performance data are analyzed by FP-growth association rules, and then clustered by K-means, which can improve the accuracy of data evaluation.The evaluation index system of university English teaching quality under the concept of “Thinking and Government” is established. With the results of the sample survey, the main problems of the evaluation method are summarized and analyzed, and corresponding suggestions are put forward, which provide an important reference for promoting the reform of college English course.
Depression is a clinical disease, mainly accompanied by mood or emotional abnormalities, mainly depression, slow thinking, often accompanied by emotional abnormalities, cognitive behavior, psychophysiological and interpersonal changes or disorders. Here, using static and task-state MRI data, we present a comprehensive study of abnormal neural activity in patients with depression through spatiotemporal, static, and dynamic measures, demonstrating its validity as an underlying biological trait. In order to effectively study the role of emotion regulation in depression, a brain dynamic network synthesis method based on support vector machine model and community detection algorithm was established. We selected data on the mental state of 45 patients from a hospital’s psychiatric disease control center. They had no history of hearing impairment and normal (or corrected) vision. All procedures are agreed in writing by each participant. The results show that this method can effectively reduce the depression degree of the subjects, and the multi-level features of the integration of task activation and task regulation connection reach 81% (\(\mathrm{<}\)0.0010, surrogate test) and 83% (\(\mathrm{<}\)0.0016, surrogate test), respectively. The recovery of its depressive psychological state has a significant impact. Numerous studies have used various forms of emotional stimuli to reveal abnormal behaviors and neural responses in multi-channel emotional processing in patients with depression, providing valuable insights into the mechanism of multi-channel emotion regulation in depression.
In order to provide users with better recommendations, it is particularly important to analyze the behavior of users tagging different resources. In this paper, an attention mechanism based on deep learning is designed to effectively capture the features related to the user’s long-term interests and current interests in the session simultaneously, and alleviate the impact of the user’s interest drift that is difficult to deal with by the current session recommendation algorithm on the recommendation accuracy. The main community discovery algorithms are applied to the clustering analysis of the label system, and their performances on different data sets are compared. Besides, we design a personalized recommendation algorithm for the label system. The experimental results show that the proposed algorithm can find the interests of different users and improve the quality of the recommendation system.
Nowadays, people look at a brand not only to see the value of the brand itself, but to understand the cultural value conveyed behind the brand and experience the connotation of the brand culture. Human-computer interaction technology has also gained more application space with the development of the times. Therefore, a psychological model of brand culture based on human-computer interaction was designed in this paper, and a survey of related content was conducted. In terms of user satisfaction survey, it was concluded that the use of the brand culture mental model based on human-computer interaction technology could greatly improve users’ satisfaction with brand culture and make more people love brand culture; in terms of user participation survey, it was concluded that the brand culture mental model designed based on human-computer interaction technology could achieve better profit results at 21:00 on Sunday. Finally, a survey of user stickiness was carried out, and the test results showed that the brand culture mental model based on human-computer interaction technology established the stickiness between users and brands.
1970-2025 CP (Manitoba, Canada) unless otherwise stated.