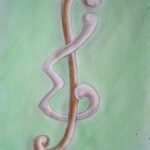
A subset
Let
The Mostar index
Green construction is becoming a mainstream model of the transformation and upgrading of the construction industry, which has the advantages of energy saving, environmental protection and ecology, which can effectively reduce energy deficiency and improve environmental quality, which is the need for high quality sustainable development. This study is based on BIM software and the intelligent construction technology to propose the green architectural design party case. Building energy-saving efficiency evaluation system, using fuzzy Borda method and the CRITIC method of evaluation, the objective of the index, and the example of a community, the use of the object meta-effect model. The evaluation scores of the energy saving efficiency of the building of green energy saving and renovation are in the 90.11-99.28 points, and the high energy demand in the process of running the use of the building is excellent in the heating, refrigeration and other aspects of the building. This paper shows that the goal of the green transformation project is basic, which is effective and the efficiency of energy efficiency is generated. This study can provide guidance for the work of the green building energy saving and renovation work, and further promote the energy saving and transformation of China.
The supply chain applies large number according to the technology, can reduce the cost of each link, optimize the resource allocation, increase the enterprise benefit. In this paper, the supply chain cost control program based on large number according to the previous forecast, the control of the event and the analysis of the three levels of the analysis of the supply chain. The combination time series model and the multivariate regression model, the joint CPFR concept, the establishment of the CPFR sales combination demand prediction model, the design form according to the sales prediction system, the resource optimization plan of the supply chain inventory in real time. Analyze the prediction effect of the combination prediction model, predict the product sales in the week, calculate the product safety inventory and the remaining inventory. The analysis is based on the cost control effect of the enterprise supply chain according to the sales forecast. The cost of purchasing the supply chain costs less than the operating income, which fell to 0.5107in 2023. The gross margin of gross profit was 0.53666 in 2023, which was controlled by the gross margin, and the gross profit was improved. It is said that the enterprise is using large number according to the technology to the supply chain resource optimization in the supply chain cost control, the cost control effect is better.
The research of modular and personalized balance strategy in assembly building design can improve the efficiency of construction and meet the demand of design diversification. Based on bim technology, an assembly building modular design method is proposed to determine the required space module, to determine the required space module, to strengthen the module structure, to set up the layout of the building, to formulate the modular panel and the assembly frame platform, and through the revit implementation of the three-dimensional visual design of modularity and personalization. The design of the 9 building of this article, in the collaborative function, spatial adaptability and the design diversity score in turn for 10th 10 “10” 9 points (full score 10). This article is designed to meet the demand of the building in daylighting and ventilation, the average daylighting coefficient is 6.440%, and the minimum value of the floor area of the building room is 18.25cent. Modular and personalized assembly frame structures have a better seismic resistance, and their limit cumulative energy consumption is 2.38 times the traditional way. Experts have the highest social benefit satisfaction in this article strategy, and the satisfaction score is 92.05.
Based on the definition of volatility and conditional value risk (CVaR), this paper introduces the implied volatility into CVaR model, and further analyzes the partial differential equation of stock portfolio optimization in the form of BS model. In the process of multi-stage investment, in order to reasonably control the investment risk of each stage, the CvaR model based on implied volatility is constructed by using the scenario tree method. With the data of 1166 trading days as the data, 4 stock assets as the data set of this study, the optimization model is applied to the calculation and analysis. The numerical simulation shows that the stock price fluctuation of the four multi-cycle stocks ranges from -23.45% to 41.97%, showing a clustering phenomenon. Among them, the volatility of stocks A and C is more obvious than that of stocks B and D, and the probability density tails of stocks are longer in the cycle, and they all show thick tail characteristics, indicating that the introduction of implied volatility of CVaR model makes the risk control of actual equity asset investment more reasonable.
In this paper, the characteristics and distribution of the spatial clustering diffusion characteristics and distribution of the spatial accumulation of rural areas are quantified by using the GIS space analysis method, the analysis method of the nuclear density estimation, the hotspot analysis, the spatial self-correlation, and the large number of the rural areas of Chongqing. Compared with the difference of the amount of the education facility in Chongqing, the difference between the amount of the education facility was compared, and the development gap of the education facility was assessed. The study showed that in 2023, the imbalance coefficient of the school of compulsory education in Chongqing was reduced from 0.3637 in 2013 to 0.02433 in 2023, and the primary school stage was reduced from 0.3582 to 0.1952. This paper shows that the imbalance coefficient of education resource layout in Chongqing is decreasing year by year, and the spatial equilibrium of resource space increases. This study provides the effective thinking and method for the adjustment of the education resource space layout structure in Chongqing, and provides the scientific decision basis for the calibration of the existing planning and the formulation of future planning.
This paper discusses the appreciation of the elderly to influence the actual exchange rate by using the requirements structure and the current account mechanism. Using the internal actual exchange rate formula and the Balassa-Samuelson effect, the propagation mechanism of the aging of the population was established. This paper discusses the influence of aging on trade balance, and sets up the panel model of countries of different age categories. Through heterogeneity analysis and multivariate regression test assessment. The study of mathematical methods found that the rate of pension care significantly affected the actual effective exchange rate. In countries where aging and moderate aging lead to depreciation, aging and non-ageing countries can rise.
In this paper, the basic Wiener filter structure and adaptive algorithm module are used to optimize the parameter adjustment and data noise processing in the adaptive filter algorithm. Based on the LMS criterion, the algorithm is further refined by quantization error and affine projection optimization, which improves the accuracy and speed of vortex and circulation data analysis. The optimized algorithm reduces noise and covariance error, and achieves excellent performance in filtering evaluation (SRTAE:
Cultural heritage represents the historical and cultural achievements of a nation, playing a vital role in studying human civilization and preserving national languages and scripts. This study utilizes virtual simulation technology to design a virtual pavilion for Chinese language and writing, employing image and text feature extraction algorithms for feature fusion and 3D modeling. The effectiveness of Chinese character extraction is validated through feature point matching, while the virtual exhibition’s impact is assessed via user experience scores. Results indicate that the proposed algorithm achieves accurate extraction with no misrecognition. User interest rankings highlight text images as the most influential factor, followed by visual imagery, pavilion experience, scene art, and language culture. Analysis of user feedback shows an average experience score exceeding 60 points, confirming the pavilion’s effectiveness in preserving and promoting Chinese language and writing culture.
In recent years, due to the adjustment of economic structure, the people’s living standard and the increase of leisure time, the sports industry has become a new economic growth point. This paper studies and analyzes the characteristics of the industry background and business background of the sports industry, explores the factors and internal driving force affecting the design of its business model, and fully analyzes the mechanism, functional role, and logical relationship of the elements for constructing the business model of the sports industry, and then explores the characteristics of the business style of the sports industry. From the perspective of knowledge state, using the reinforcement learning mechanism, the evolution process of the sports industry business model from the first stage to the fourth stage is described. Taking Company H as a research case, the process and economic effect of the transformation and upgrading of its business model through the reinforcement learning mechanism is analyzed and it is found that as of 2023 the company’s operating income has increased by 2.4 times through transformation and upgrading, and its net profit has increased by 125.57 percentage points compared to 2016. It further understands the role that the enhanced learning mechanism brings to the development of the sports industry, and expects to be able to provide a reference for the sports industry to carry out business model transformation in the future.
We initiate a study of the toughness of directed graphs by considering the natural generalization of that for ordinary graphs. After providing some general results, computations are completed for a few natural examples. Maximum possible toughness is also considered. Some open problems are posed.
Let
This study applies Support Vector Machine (SVM) and K-Nearest Neighbors (KNN) algorithms to classify five types of basketball footwork. SVM maps the training data into a high-dimensional space using nonlinear transformation and classifies it with support vectors and a hyperplane. Experimental analysis showed minimal differences in peak and trough values of footwork movements; therefore, only mean and standard deviation features were retained, resulting in 12 effective features. KNN experiments demonstrated that recognition accuracy varies with different K values. The highest accuracy (80.7%) was achieved when K = 5 with the selected features. The study also examined the physical characteristics of basketball players, analyzing height, weight, and other indicators. Statistical results showed no significant body shape differences between experimental and control groups (P > 0.05). A T-test on dribbling, shooting, and layup performance also revealed no significant differences between the groups (P > 0.05).
This study explores the employment competitiveness of computer science majors by integrating combinatorial mathematics into the evaluation process. Utilizing the Analytic Hierarchy Process (AHP) and the improved FKCM clustering algorithm, we construct a hierarchical model to assess the impact of entrepreneurial education, learning motivation, and investment on job competitiveness. Data from 314 participants were analyzed using combinatorial techniques to derive optimal weightings for each factor, ensuring the evaluation model’s robustness. The results highlight significant gender differences in practical and feedback-based entrepreneurship education, with males outperforming females. However, no notable differences were observed in job interest, learning motivation, or overall employment competitiveness.
An (unrooted) binary tree is a tree in which every internal vertex has degree
This paper proposes an optimized Backpropagation (BP) neural network for improving intelligent elderly care talent training. To address BP’s limitations, including noise sensitivity and slow convergence, we introduce Particle Swarm Optimization (PSO) to refine network weights and thresholds. The model integrates course quality, teacher effectiveness, platform support, and market demand, aiming to optimize elderly care service talent cultivation. Experimental results demonstrate a significant improvement in prediction accuracy, with average error reduced from 9.94% to 6.3%. This enhanced model offers a more efficient and accurate solution for aligning educational outcomes with industry needs.
Amnesty international is recognized as a key force in promoting social development, with higher education also facing the need for innovation. This paper explores new opportunities in educational theory and policy proposed in a recent initiative. The proposal emphasizes filtering ideology, political education, and public opinion to enhance the accuracy of ideological and political teaching. By incorporating personal suggestions through interviews, the model recommends learning materials tailored to student characteristics. System implementation and testing demonstrate its potential as a core tool for ideological education in colleges, supporting the integration of knowledge, politics, and technology to meet students’ educational needs.
Networks with smaller strong diameters generally have better fault tolerance because they enable closer connections between vertices, leading to shorter information paths. This allows the network to maintain communication and functionality more effectively during attacks or failures. In contrast, larger strong diameters mean vertices are connected over longer distances, increasing vulnerability to disruptions. Thus, the strong diameter is a key metric for assessing and optimizing network fault tolerance. This paper determines the optimal orientations for the Cartesian and strong products of even cycles, provides the minimum strong diameters and their bounds under specific conditions, and establishes a lower bound for the maximum strong diameter. A conjecture about the exact value of the maximum strong diameter is also proposed.
For a graph
The development of artificial intelligence enables computers to not only simulate human artistic creations, but also synthesize fine art works with deeper meanings based on natural images. This study digitally parses the fusion of fine art and philosophy visual expressions, and develops a visual expression system based on the fusion of fine art and philosophy by utilizing a variety of key big data algorithms for visual expressions such as adversarial networks. Research on pattern recognition of this system in art creation is carried out through model training, recommendation performance evaluation, pattern recognition strategy application and regression analysis. The model in this paper works best when the number of nearest neighbors k=15, and the recommendation model in this paper can provide a personalized list of artwork recommendations for different people. The recognition of the system in this paper in the five dimensions of “spiritual level”, “value level”, “philosophical level”, “aesthetic level” and “technical level” is distributed between 4.24
With development of Internet of Things, big data and artificial intelligence, cell phone signaling data, point-of-interest data and machine learning methods have been widely used in research of various fields of transportation. The use of big data processing techniques and machine learning methods to mine intercity travel data collected by various types of traffic detectors provides a new way of thinking to study travel mode selection behavior. In this paper, we pre-processed cell phone signaling data, geospatial data and interest point data around three aspects: personal attributes, travel attributes and travel mode attributes, and designed intercity travel target group extraction, travel chain extraction, travel mode extraction and travel purpose extraction algorithms, which provide basis for travel feature analysis and travel mode choice behavior prediction modeling.
Conventional techniques to electric power network (EPN) design and management are insufficient to handle extreme weather events like hurricanes due to the growing complexity and fragility of power systems. As a sophisticated simulation and optimization tool, digital twin (DT) technology may offer real-time power infrastructure monitoring and prediction. This study aims to investigate the possible application of digital twin technology in enhancing power system resilience and streamlining the design process, as well as to use it for the 3D design of the full substation engineering infrastructure process. A digital twin-based EPN model that incorporates all of the main components of the power system—power plants, substations, transmission and distribution networks, and customers—is proposed in this paper. Every component of the power system undergoes vulnerability analysis, and the chance of the system failing is calculated using a Bayesian network (BN) model and a parametric vulnerability function. According to modeling projections, Hurricane Ike will cause the majority of consumers’ power supplies to be interrupted. The model predicts that power consumption for residential, commercial, and industrial buildings will be 96.4%, 96.0%, and 94.2%, respectively, depending on the kind of building.
Radar ranging and speed measurement are common applications in daily life, with performance largely dependent on the radar signal processor. However, existing civilian radar signal processors struggle with weak signal reception and low analysis efficiency. This study designs a high-speed radar signal processor based on FPGA architecture, incorporating a fusion processing algorithm to integrate different radar signal bands, enhancing processing efficiency and accuracy. The design includes data feature analysis, storage, and fusion modules. Tests showed that the processor achieved real-time performance with a processing time under 1ms, a ranging error below 1m, and speed measurement accuracy within 5m/s, meeting practical requirements.
Intriguing symmetries are uncovered regarding all magic squares of orders 3, 4, and 5, with 1, 880, and 275,305,224 distinct configurations, respectively. In analogy with the travelling salesman problem, the distributions of the total topological distances of the paths travelled by passing through all the vertices (matrix elements) only once and spanning all elements of the matrix are analyzed. Symmetries are found to characterize the distributions of the total topological distances in these instances. These results raise open questions about the symmetries found in higher-order magic squares and the formulation of their minimum and maximum total path lengths.
In this paper, we introduce the concept of vertex-edge locating Roman dominating functions in graphs. A vertex-edge locating Roman dominating
Traditional personnel recruitment methods are often inefficient and struggle to find candidates who meet job requirements. In this paper, we first develop a comprehensive personnel management system for colleges and universities that streamlines the recruitment process and information management. Next, recruitment data from the system is analyzed using the fuzzy C-means algorithm to cluster applicant profiles and extract position-specific user characteristics. Finally, a joint embedded neural network is employed to match applicant profiles with job positions by optimizing an objective function. Experimental results demonstrate a high job matching rate (up to 98.1%), a significantly reduced recruitment cycle (from job posting to candidate onboarding in 25 days), and a system response time as low as 0.5 seconds. These findings highlight the effectiveness of big data technology in providing timely feedback, reducing recruitment costs and staff workload, and promoting the intelligent development of talent recruitment.
The rapid development of information technology makes intelligent decision support system play an increasingly important role in economic standardized management. The Intelligent Decision Support System (IDSS) constructed in this paper includes interaction layer, analysis layer and data layer. The system standardizes the management of enterprise economy through strategic forecasting and decision analysis, economic planning and control, and economic analysis. The study combines the fuzzy hierarchical analysis method (FAHP) and the fuzzy comprehensive evaluation method (FCE) to evaluate the standardized level of economic management of enterprise A. The evaluation score of the standardized level of enterprise A’s economic management is
In order to solve the multi-objective optimization problem of resource allocation in enterprise strategic management, the article firstly establishes a multi-objective resource allocation model for maximizing the benefits of enterprises in enterprise strategic management. Then, it optimizes and improves the initial population, convergence factor and dynamic weights of the gray wolf algorithm, increases the population diversity by using the population strategy of reverse learning, improves the convergence factor into a nonlinear factor, and finally changes the decision-making weights of the gray wolf leadership and applies the dynamic weights to improve the accuracy of the algorithm. Subsequently, the improved gray wolf algorithm is utilized for model decoupling. By applying this paper’s algorithm and the other two algorithms to solve the six algorithms 30*6, 60*6, 90*2, 90*4, 150*4 and 150*6 for 9 times, it is found that in the analysis of the 30*6 algorithm, the enterprise’s resource allocation reaches 5,000 when the time is 110 s. At the same time, this paper’s algorithm obtains a better non-dominated solution than the other two algorithms, which proves that this paper’s algorithm solves the multi-objective resource allocation problem of enterprise law industry is proved to be effective.
In food processing, foreign matter inevitably contaminates packaged food. To ensure food safety, ray-based detection is used; however, the original images suffer from aberrations and noise that degrade quality and hinder further processing. Thus, images are preprocessed to enhance quality by highlighting key features and suppressing irrelevant ones before abnormal pattern recognition. Following image segmentation, a BP neural network algorithm is applied for foreign object detection. In tests with contaminants such as metal wires, stones, and glass, the algorithm identified distinct abnormal fluctuations at gray levels of 132, 108, and 34, respectively, allowing it to reliably detect foreign objects. Although the practical detection rate reached 100%, occasional misjudgments suggest that further optimization is needed. Overall, this method introduces a novel approach to detecting foreign objects in food and offers promising new strategies for improving food safety monitoring.
1970-2025 CP (Manitoba, Canada) unless otherwise stated.