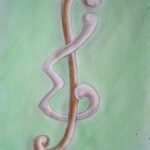
In this paper, it is pointed out that the definition of `Fibonacci \((p,r)\)-cube’ in many papers (denoted by \(I\Gamma_{n}^{(p,r)}\)) is incorrect. The graph \(I\Gamma_{n}^{(p,r)}\) is not the same as the original one (denoted by \(O\Gamma_{n}^{(p,r)}\)) introduced by Egiazarian and Astola. First, it is shown that \(I\Gamma_{n}^{(p,r)}\) and \(O\Gamma_{n}^{(p,r)}\) have different recursive structure. Then, it is proven that all the graphs \(O\Gamma_{n}^{(p,r)}\) are partial cubes. However, only a small part of graphs \(I\Gamma_{n}^{(p,r)}\) are partial cubes. It is also shown that \(I\Gamma_{n}^{(p,r)}\) and \(O\Gamma_{n}^{(p,r)}\) have different medianicity. Finally, several questions are listed for further investigation.
A \(q\)-total coloring of \(G\) is an assignment of \(q\) colors to the vertices and edges of \(G\), so that adjacent or incident elements have different colors. The Total Coloring Conjecture (TCC) asserts that a total coloring of a graph \(G\) has at least \(\Delta+1\) and at most \(\Delta+2\) colors. In this paper, we determine that all members of new infinite families of snarks obtained by the Kochol superposition of Goldberg and Loupekine with Blowup and Semiblowup snarks are Type~1. These results contribute to a question posed by Brinkmann, Preissmann and D. Sasaki (2015) by presenting negative evidence about the existence of Type~2 cubic graphs with girth at least 5.
Generative adversarial network (GAN) technology has enabled the automatic synthesis of realistic face images from text. This paper proposes a model for generating face images from Chinese text by integrating a text mapping module with the StyleGAN generator. The text mapping module utilizes the CLIP model for pre-training Chinese text, employs a convolutional-inverse convolutional structure to enhance feature extraction, and incorporates a BiLSTM model to construct complete sentences as inputs for the StyleGAN generator. The generator interprets semantic features to generate face images. Validation on Face2Text and COCO datasets yields F1 values of 83.43% and 84.97%, respectively, while achieving the lowest FID and FSD scores of 103.25 and 1.26. The combination of CLIP pre-training and word-level semantic embedding improves image quality, offering a novel approach for face recognition applications in public safety.
In this note, we establish six Gallai theorems involving twelve minority and majority parameters. Accordingly, the complexity problems corresponding to some of these parameters are obtained.
The promotion of industrial digital transformation is a crucial breakthrough in the evolution of economic structures and the physical layout of spaces. It has the potential to elevate the entire industrial chain to a high-end value chain, creating more profit opportunities and enhancing the influence of domestic industries in the international cycle. This study uses the cities in the Yangtze River Delta Economic Belt as a case study to explore the spatial effects of digital transformation on the healthy transformation of traditional industrial structures. It constructs relevant spatial coupling models and empirically verifies them by testing specific assumptions. The experimental results indicate that the model is significant at a level greater than 5%, making it suitable for selecting spatial measurement models. The mean square error of its network simulation output is 0.1333, confirming the expected hypothesis and demonstrating that digital transformation has a significant spatial driving effect on industrial upgrading.
A \(k\)-tree is a graph that can be formed by starting with \(K_{k+1}\) and iterating the operation of making a new vertex adjacent to all the vertices of a \(k\)-clique of the existing graph. A structural characterization of 3-trees with diameter at most 2 is proven. This implies a corollary for planar 3-trees which leads to a description of their degree sequences.
Electric shock accidents remain a major safety concern for distribution workers. Recent advancements in video AI applications allow for detecting when workers cross safety lines, but determining their height and the spatial distance between them and live equipment is still a challenge. This article proposes a pre-control system using LiDAR, an edge processing module, and a warning module to ensure safe operations in power distribution scenarios. The system scans the area in real time, uses deep learning to identify objects like distribution stations, human bodies, high-voltage equipment, and transmission lines in point clouds, and calculates the distance between operators and high-voltage equipment. When this distance approaches or exceeds safety limits, the warning module issues voice alerts. Experimental results show that this system significantly reduces false alarms compared to video-based methods, accurately measures distances, and provides timely warnings, making it a practical solution for enhancing worker safety in power distribution operations.
In this paper, we present a new combinatorial characterization of Hermitian cones in \(\mathrm{PG}(3,q^2)\).
Let \(K_n\), \(P_n\), and \(Y_n\) respectively denote a complete graph, a path, and a \(Y\)-tree on \(n\) vertices, and let \(K_{m,n}\) denote a complete bipartite graph with \(m\) and \(n\) vertices in its parts. Graph decomposition is the process of breaking down a graph into a collection of edge-disjoint subgraphs. A graph \(G\) has a \((H_1, H_2)\)-multi-decomposition if it can be decomposed into \(\alpha \geq 0\) copies of \(H_1\) and \(\beta \geq 0\) copies of \(H_2\), where \(H_1\) and \(H_2\) are subgraphs of \(G\). In this paper, we derive the necessary and sufficient conditions for the \((P_5, Y_5)\)-multi-decomposition of \(K_n\) and \(K_{m,n}\).
With the rapid development of wireless communication networks, it brings more and more convenience to users. However, with the expansion of network size, the limitation of channel resources in network communication is becoming more obvious. Effective channel assignment has a great impact on the quality of communication networks. However, in real communication networks, underutilization of channels and excessive number of channels produce large interference, so it is necessary to find a reasonable channel assignment method. In this paper, we study the optimal channel assignment strategy for the Cartesian product of an \(m\)-vertex complete bipartite graph and an \(m\)-order cycle, where \(m\geq 5\) is odd. Determines the exact value and lower bound of its radio number.
This study introduces a novel approach to investigating Sombor indices and applying machine learning methods to assess the similarity of non-steroidal anti-inflammatory drugs (NSAIDs). The research aims to predict the structural similarities of nine commonly prescribed NSAIDs using a machine learning technique, specifically a linear regression model. Initially, Sombor indices are calculated for nine different NSAID drugs, providing numerical representations of their molecular structures. These indices are then used as features in a linear regression model trained to predict the similarity values of drug combinations. The model’s prediction performance is evaluated by comparing the predicted similarity values with the actual similarity values. Python programming is employed to verify accuracy and conduct error analysis.
Criminal evidence serves as the foundation for criminal proceedings, with evidence used to ascertain the facts of cases being critical to achieving fairness and justice. This study explores the application of digital information technology in building a data resource base for criminal cases, formulating standard evidence guideline rules, and optimizing evidence verification procedures. A named entity recognition model based on the SVM-BiLSTM-CRF framework is proposed, coupled with an evidence relationship extraction model using the Transformer framework to improve evidence information extraction through sequential features and global feature capturing. Results show that the F1 value for entity recognition in criminal cases reaches 94.19%, and the evidence extraction model achieves an F1 value of 81.83% on the CAIL-A dataset. These results are utilized to construct evidence guidelines, helping case handlers increase case resolution rates to approximately 99%. The application of digital technology enhances evidence collection efficiency, accelerates case closures, and offers a pathway to improving judicial credibility.
In this paper we consider some new weighted and alternating weighted generalized Fibonomial sums and the corresponding \(q-\)forms. A generalized form of weight sequences which contains squares in subscripts is discussed for the first time in the literature. The main key to get success in sums is an ability to change one sum into another that is simpler in some way. Thus, in order to prove these sums by doing some manipulations and tricks, our approach is to use classical \(q-\)analysis, in particular a formula of Rothe, a version of Cauchy binomial theorem and Gauss identity.
Total dominator total coloring of a graph is a total coloring of the graph such that each object of the graph is adjacent or incident to every object of some color class. The minimum namber of the color classes of a total dominator total coloring of a graph is called the total dominator total chromatic number of the graph. Here, we will find the total dominator chromatic numbers of wheels, complete bipartite graphs and complete graphs.
Meta-analysis was conducted to investigate the effects of static versus dynamic stretching on athlete agility. Keywords such as dynamic stretching, static stretching, athletes, and agility were searched through China Knowledge Network (CNKI), Wanfang, Pubmed, Web of Science, and EBSCO. Inclusion and exclusion criteria were established, and Endnote software was used to screen the literature, with statistical analysis performed using Stata and Revman. A total of 15 papers with 322 groups of experiments were included, with interventions typically performed three times a week. The quality of the included papers, assessed using Review Manager, showed all studies to be randomized controlled trials with low-risk indicators. Meta-analysis results indicated high heterogeneity with SMD=0.11 and significant differences (P<0.00001<0.05). The findings suggest that static and dynamic stretching, with an intervention period of about 15 weeks and a frequency of approximately three times per week, have a significant effect on athlete agility.
A new series of four-associate class partially balanced incomplete block designs in two replications has been proposed. The blocks of these designs are of two different sizes. The blocks can be divided into two groups such that every treatment appears in each group exactly once, and any two blocks belonging to two different groups have a constant number of treatments in common, i.e., these designs are affine resolvable.
We initiate to study a \(D\)-irregular labeling, which generalizes both non-inclusive and inclusive \(d\)-distance irregular labeling of graphs. Let \(G=(V(G),E(G))\) be a graph, \(D\) a set of distances, and \(k\) a positive integer. A mapping \(\varphi\) from \(V(G)\) to the set of positive integers \(\{1,2,\dots,k\}\) is called a \(D\)-irregular \(k\)-labeling of \(G\) if every two distinct vertices have distinct weights, where the weight of a vertex \(x\) is defined as the sum of labels of vertices whose distance from \(x\) belongs to \(D\). The least integer \(k\) for which \(G\) admits a \(D\)-irregular labeling is the \(D\)-irregularity strength of \(G\) and denoted by \(\mathrm{s}_D(G)\). In this paper, we establish several fundamental properties on \(D\)-irregularity strength for arbitrary graphs. We also determine this parameter exactly for families of graphs with small diameter or small maximum degree.
Let \( 0<k\in\mathbb{Z} \). Let the star 2-set transposition graph \( ST^2_k \) be the \( (2k-1) \)-regular graph whose vertices are the \( 2k \)-strings on \( k \) symbols, each symbol repeated twice, with its edges given each by the transposition of the initial entry of one such \( 2k \)-string with any entry that contains a different symbol than that of the initial entry. The pancake 2-set transposition graph \( PC^2_k \) has the same vertex set of \( ST^2_k \) and its edges involving each the maximal product of concentric disjoint transpositions in any prefix of an endvertex string, including the external transposition being that of an edge of \( ST^2_k \). For \( 1<k\in\mathbb{Z} \), we show that \( ST^2_k \) and \( PC^2_k \), among other intermediate transposition graphs, have total colorings via \( 2k-1 \) colors. They, in turn, yield efficient dominating sets, or E-sets, of the vertex sets of \( ST^2_k \) and \( PC^2_k \), and partitions into \( 2k-1 \) such E-sets, generalizing Dejter-Serra work on E-sets in such graphs.
The scientific knowledge graph is an emerging research method in this context. In the research of physical education teaching, the research and sorting out of the research results of physical education teaching in my country from the perspective of scientometrics and information visualization is still slightly insufficient. The similarity between the frontiers of physical education teaching research in China and the United States in the past five years is that both countries have paid more attention to research topics such as physical education teaching methods and physical education courses. This paper proposes a rough set knowledge reduction algorithm based on improved genetic algorithm. The support and importance of conditional attributes to decision attributes are introduced into the information system, which are added to the genetic algorithm as heuristic information, and the concepts of population dissimilarity and individual dissimilarity are proposed to improve the genetic algorithm. The research on school physical education in my country is biased towards problem research, while the research on physical education teaching methods in the United States is biased towards student health; In addition, starting from the national conditions, the hotspots in the field of physical education teaching in my country tend to be “Sports and Health Curriculum Standards”, physical education teachers, physical education ideas, educational theories and college sports, while the hotspots in the field of physical education teaching in the United States tend to be physical activity, children and adolescents , students, women, exercise education, physical education, self-determination theory, and the integration of psychological motivation and physical education. Experimental data analysis my country’s physical education curriculum research should appropriately increase the attention to the details of physical education curriculum, and my country’s physical education teaching practice research should appropriately increase the research on physical education from the perspective of public health.
In secret sharing, the relationships between participants and the information they hold can be modeled effectively using graph structures. Graphs allow us to visualize and analyze these relationships, making it easier to define access structures, optimize share distributions, and ensure security. This paper provides the first comprehensive review of existing research on the application of graph theory to secret sharing comparing different classic and modern approaches and analyzing the current litterature. Through this study we highlight the key advances and methodologies that have been developed, underscoring the pivotal role of graph theoretic approaches in enhancing the security and efficiency of secret sharing schemes. Furthermore, the review identifies open challenges and future research directions, providing insights into potential innovations that could further strengthen cryptographic practices. This work serves as a foundational resource for researchers and practitioners seeking to deepen their understanding of the intersection between graph theory and secret sharing, fostering the development of more robust and sophisticated cryptographic solutions.
A proper coloring assigns distinct colors to the adjacent vertices of a graph. An equitable near proper coloring of a graph \(G\) is an improper coloring in which neighbouring vertices are allowed to receive the same color such that the cardinalities of two distinct color classes differ by not more than one and the number of monochromatic edges is minimised by giving certain restrictions on the number of color classes that can have an edge between them. This paper discusses the equitable near proper coloring of line, middle, and total graphs of certain graph classes, such as paths, cycles, sunlet graphs, star graphs, and gear graphs.
This paper investigates the Turan-like problem for \(\mathcal{K}^-_{r + 1}\)-free \((r \geq 2)\) unbalanced signed graphs, where \(\mathcal{K}^-_{r + 1}\) is the set of unbalanced signed complete graphs with \(r+1\) vertices. The maximum number of edges and the maximum index for \(\mathcal{K}^-_{r + 1}\)-free unbalanced signed graphs are given. Moreover, the extremal \(\mathcal{K}^-_{r + 1}\)-free unbalanced signed graphs with the maximum index are characterized.
Directed hypergraphs represent a natural extension of directed graphs, while soft set theory provides a method for addressing vagueness and uncertainty. This paper introduces the notion of soft directed hypergraphs by integrating soft set principles into directed hypergraphs. Through parameterization, soft directed hypergraphs yield a sequence of relation descriptions derived from a directed hypergraph. Additionally, we present several operations for soft directed hypergraphs, including extended union, restricted union, extended intersection, and restricted intersection, and explore their characteristics.
In this paper, we give a classification of all Mengerian \(4\)-uniform hypergraphs derived from graphs.
Big data technology makes it possible to scientifically analyse a large amount of marketing data, which plays an important role in the development of marketing strategies for products and the improvement of marketing effects. In this paper, a marketing data stream analysis system is designed based on the stream analysis method. The system designs and optimises the marketing data storage and retrieval, data acquisition and streaming calculation engine to achieve real-time user behaviour data streaming analysis. The average response time accuracy of the system’s data can reach 96%, the throughput rate is 11.8% ahead of the maximum compared to the Word Count system, and the before-and-after ratios of the PUSH message click rate, the user registration success rate, the online shop attention rate, the returning customer rate, and the loyal customer rate are 1.03, 1.02, 1.27, 1.11, 1.27, and 1.78, respectively. It indicates that this paper’s design of the marketing data streaming analysis system has good performance and application effect.
With the construction of the national discourse power, the international communication of German language has also attracted the attention of the public, and its own communication attributes and characteristics have also become a hot topic around the world. A machine learning development process includes operations such as data preprocessing, feature engineering, model design, and super parameter optimization. Changes in the configuration of each operation may affect the final quality of the model. Nor is it mainly the problem of teachers’ teaching, but the communication barrier caused by cultural differences. We can see that there are still many obstacles and misunderstandings in language, thought, cross-cultural communication and knowledge in many communication occasions between China and Germany. Through reviewing and summarizing the previous studies on intercultural communication, this paper analyzes the current situation of intercultural communication studies, points out the problems existing in the current research, and tries to put forward the cultivation methods of intercultural communication.
The \( n \)-dimensional Möbius cube \( MQ_n \) is an important variant of the hypercube \( Q_n \), which possesses some properties superior to the hypercube. This paper investigates the fault-tolerant edge-pancyclicity of \( MQ_n \), and shows that if \( MQ_n \) (\( n \geq 5 \)) contains at most \( n-2 \) faulty vertices and/or edges then, for any fault-free edge \( uv \) in \( MQ_n^i (i=0,1) \) and any integer \( \ell \) with \( 7-i \leqslant \ell \leqslant 2^n – f_v \), there is a fault-free cycle of length \( \ell \) containing the edge \( uv \), where \( f_v \) is the number of faulty vertices. The result is optimal in some senses.
For a connected graph \(G\), the edge Mostar index \(Mo_e(G)\) is defined as \(Mo_e(G)=\sum\limits_{e=uv \in E(G)}|m_u(e|G) – m_v(e|G)|\), where \(m_u(e|G)\) and \(m_v(e|G)\) are respectively, the number of edges of \(G\) lying closer to vertex \(u\) than to vertex \(v\) and the number of edges of \(G\) lying closer to vertex \(v\) than to vertex \(u\). We determine a sharp upper bound for the edge Mostar index on bicyclic graphs and identify the graphs that achieve the bound, which disproves a conjecture proposed by Liu et al. [Iranian J. Math. Chem. 11(2) (2020) 95–106].
In a recent paper Cameron, Lakshmanan and Ajith [6] began an exploration of hypergraphs defined on algebraic structures, especially groups, to investigate whether this can add a new perspective. Following their suggestions, we consider suitable hypergraphs encoding the generating properties of a finite group. In particular, answering a question asked in their paper, we classified the finite solvable groups whose generating hypergraph is the basis hypergraph of a matroid.
Given a connected graph \(G\) and a configuration \(D\) of pebbles on the vertices of \(G\), a pebbling transformation involves removing two pebbles from one vertex and placing one pebble on its adjacent vertex. A monophonic path is defined as a chordless path between two non-adjacent vertices \(u\) and \(v\). The monophonic cover pebbling number, \(\gamma_{\mu}(G)\), is the minimum number of pebbles required to ensure that, after a series of pebbling transformations using monophonic paths, all vertices of \(G\) are covered with at least one pebble each. In this paper, we determine the monophonic cover pebbling number (\(MCPN\)) for the gear graph, sunflower planar graph, sun graph, closed sun graph, tadpole graph, lollipop graph, double star-path graph, and a class of fuses.
Chinese animation has long faced challenges, with foreign animation dominating the market and domestic animation struggling to compete. The rise of new media has driven the industrialization and branding of Chinese animation, linking it to complex social and cultural networks that shape its future competitiveness. Similarly, sports events, as cultural phenomena, hold both entertainment and cultural significance, reflecting societal modernization. This study categorizes mascot design features of sports events into appearance, color, and accessory characteristics, providing theoretical insights to enhance understanding of event culture. Experimental results show that an optimized cellular genetic algorithm improves mascot design, aligning with human aesthetics while promoting the spirit of sports globally.
By means of the generating function method, a linear recurrence relation is explicitly resolved. The solution is expressed in terms of the Stirling numbers of both the first and the second kind. Two remarkable pairs of combinatorial identities (Theorems 3.1 and 3.3) are established as applications, that contain some well–known convolution formulae on Stirling numbers as special cases.
A \(\mathcal{Y}\) tree on \(k\) vertices is denoted by \(\mathcal{Y}_k\). To decompose a graph into \(\mathcal{Y}_k\) trees, it is necessary to create a collection of subgraphs that are isomorphic to \(\mathcal{Y}_k\) tree and are all distinct. It is possible to acquire the necessary condition to decompose \(K_m(n)\) into \(\mathcal{Y}_k\) trees (\(k \geq 5\)), which has been obtained as \(n^2m(m-1) \equiv 0 \pmod{2(k-1)}\). It has been demonstrated in this document that, a gregarious \(\mathcal{Y}_5\) tree decomposition in \(K_m(n)\) is possible only if \(n^2m(m-1) \equiv 0 \pmod{8}\).
Let \( G \) be a graph, the zero forcing number \( Z(G) \) is the minimum of \( |Z| \) over all zero forcing sets \( Z \subseteq V(G) \). In this paper, we are interested in studying the zero forcing number of quartic circulant graphs \( C_{p}\left(s,t\right) \), where \( p \) is an odd prime. Based on the fact that \( C_{p}\left(s,t\right) \cong C_{p}\left(1,q\right) \), we give the exact values of the zero forcing number of some specific quartic circulant graphs.
This paper first introduces the regional power marketing management platform, after which the 3 major functional modules of this power marketing management platform are designed. Then MobileFaceNet is used as the basic network for face recognition feature extraction in the context of deep learning, and the SE module is used to optimize the network performance and network expressiveness. Afterwards, the Taylor expansion of the negative log-likelihood function is used as an optimization criterion to optimize the face detection model (MTCNN) and the face recognition model (SE-MobileFaceNet). Finally, the running effect and performance of SE-MobileFaceNet model are measured. The main conclusions are as follows: in 1:1 mode, the accuracy of SE-MobileFaceNet model for the three datasets DRDS, DE and DPDS is 95.99%, 96.98% and 98.83%, respectively. In addition, the SE-MobileFaceNet model can avoid excessive redundant calculations, so that its recognition rate reaches 95%.The accuracy of the SE-MobileFaceNet model for monitoring and recognizing the information of the management platform ranges from 97.43% to 100%, and it has a good operating effect in the identification of the regional electric power marketing management information platform, and the overall satisfaction rate of the testers for the model is also >85%. The overall satisfaction of the testers to the model is also >85%. Obviously, the SE-MobileFaceNet model proposed in this paper has a very broad application in regional power marketing management information platform identity recognition.
The emergence of digital humanities echoes the paradigm transformation of literary research. In this project, natural language processing technology is applied to the analysis of ancient Chinese literature, and the attention mechanism is embedded in iterative null convolutional network to build a named entity recognition model based on ancient literature. Then we integrate MacBERT pre-training model, combine the dual-channel structure of aspectual word features and semantic features, design hierarchical attention mechanism, and complete the construction of aspectual-level sentiment analysis model. Through experiments to analyze the model performance and conduct the analysis of ancient literary works, the results can be obtained that the two models show better named entity recognition and sentiment analysis effects, and the values of each evaluation index are above 83%. Craftsmen (44.7%) and merchants (22.4%) appear more frequently in the sample Ming Dynasty fictional characters, revealing the social and cultural background of the development of the commercial economy and the emergence of the civic class at that time. Positive emotions accounted for 67.9% of the poetry samples of the Tang Dynasty, and the war of the country’s reward (0.334) and send-off feelings (0.226) co-occurred most frequently with positive emotions, reflecting the social and cultural background of the Tang Dynasty, which was characterized by prosperity and affluence, and the emergence of the literati to build up their careers.
The development of urbanization is rapidly changing, and various undertakings are flourishing, while the sports industry, as an important segment of urban regional economic development, plays an inestimable role in the development of the entire city construction. The study takes the sports industry and economic development of 27 provincial capital cities in China from 2018 to 2022 as the research object, establishes the evaluation indexes for the high-quality development of the sports industry based on the principle of index construction, and establishes the weights of the indexes. Taking Harbin as a case study, the effect between urban sports industry and economic growth is analyzed with the help of impulse response analysis, Granger causality test, and variance decomposition of VAR model. The results show that the development of urban sports industry and economic growth can promote each other, with a long-term cointegration relationship, and the positive effects between the two are slowly reduced over time when they are impacted in the long term. Granger test shows. It indicates that there is a unidirectional causal relationship between urban sports industry and economic growth.
Flexibility control and vision of robots are important acquisition and feedback links in robot control, and the study of multi-sensor data fusion is becoming more and more important as the complexity of robot tasks increases. This paper describes the robot kinematics and inverse kinematics process by studying the knowledge of D-H model theory and parameter definitions in the machine kinematics model, reveals the changing relationship between the robot joint control and end pose, and establishes a kinematics-based vision servo control model. On this basis, the coupling error compensation algorithm is used to combine the visual position control quantity as well as the force sensing position correction quantity to form the final visual and force sensing supple control strategy. Meanwhile, for the lack of adaptability of classical impedance force control on unknown constraint environments, a two-fuzzy adaptive sliding mode controller is designed according to the Lyapunov stability theorem to drive the robot end in order to achieve the actual position tracking expectation. The results of simulation experiments and motion contour tracking experiments show that the control algorithm proposed in this paper has better control accuracy and is more robust to noise and uncertainty, and the controller is also able to reduce the effect of torque saturation on the robot system.
Garden is an important support for regional economic development, but also an important support for regional ecological environmental protection, the rational allocation of water resources in the garden is one of the effective ways to solve the problem of water shortage. This paper takes the Internet of things, digital twin as the technical basis, uses the multi-objective optimisation algorithm to construct the water resource management model of the garden area, and uses the artificial fish swarm algorithm to solve the model. By constructing a digital twin irrigation district water resources scheduling management platform, the water resources elements of the garden area are comprehensively monitored and sensed, and the intelligent simulation of the water resources allocation management process and decision-making scheme evaluation and optimisation are achieved, so as to enhance the intelligent and refined management level of water resources scheduling of the garden area, and comprehensively realise the saving and intensive use of water resources. Taking X garden area as a research case, the water resources management model finally derives the optimal water resources allocation scheme under 50%, 75% and 90% in each planning year, which provides support for the efficient use of water resources in X garden area.
This paper evaluates the quality of university English teaching based on the hierarchical analysis algorithm (AHP) and fuzzy comprehensive evaluation algorithm (FCEA) in order to grasp the teaching situation more objectively. Based on the principle of evaluation index system construction, the evaluation indexes of university English teaching quality are determined. Using hierarchical analysis algorithm to calculate the weights of its indicators, and constructing a fuzzy comprehensive evaluation matrix based on expert ratings to finalize the assessment of university English teaching quality. Taking a university as the research subject, the English teaching quality assessment result of the university is 3.7351, and its corresponding fuzzy comprehensive evaluation A=(0.2893,0.3981,0.1359,0.1120,0.0648), which summarizes the teaching quality of university English as good according to the principle of maximum affiliation degree. In order to improve the teaching of college English in this university, corresponding teaching strategies of college English are proposed.
Deep learning, as a multilayer neural network structure for deep learning of data features, can describe the nonlinear mapping relationship for the assessment of college civic education. Aiming at the current education quality assessment model based on deep learning, this paper proposes an optimized convolutional neural network (HOA-CNN) based on Hummingbird Optimization Algorithm to assess the quality of Civic and Political Education in colleges and universities. According to the correlation coefficient between the objective assessment results and the subjective assessment results, the objective assessment results of the quality of Civic and Political Education in colleges and universities are obtained. The test results show that the linear correlation coefficient and the rank correlation coefficient between the assessment results of this method and the subjective assessment results are closer to 1. The goodness-of-fit of the assessment of the quality of college civic education under the model of this paper is significantly higher than that of the two control models. The simulation test results show that the assessment results of the university civic education quality assessment model constructed by the optimized convolutional neural network based on the hummingbird optimization algorithm are more accurate.
When a steam turbine blade has cracks, fractures, or other flaws, the steam turbine’s operating circumstances will change the vibration characteristics of the blades, complicating the problem identification process. The important defect features are difficult to automatically and effectively extract from the recorded vibration signals. In this study, the input signal characteristics for a particular operating situation are used as labels to reconstruct a trained autoencoder utilizing a reverse error. The supervised autoencoder receives the fault features for various speed circumstances, which it then protectively maps to a series of reference condition features. The goal is to eliminate the disruption brought on by variations in fault feature values brought on by alterations in operating circumstances. The experimental findings demonstrate that this approach can more effectively convert feature sequences under various working situations and address the issue of fault feature distortion brought on by changes in working conditions. In addition, comparison of clustering visualization and accuracy of classification methods on data before and after commutation demonstrates that the proposed supervised autoencoder model can extract accurate classifiable features for fault classification.
The research combines PBL teaching method, CDIO theory and school-enterprise collaborative education mechanism to construct a school-enterprise collaborative teaching model based on PBL-CDIO. And then, the empirical research of PBL-CDIO school-enterprise collaborative teaching mode is realized through the teaching experiment method. The independent sample t-test is used to test the changes in the professional knowledge level and basic working ability of the experimental group and the control group before and after the experiment, and to judge the teaching effect of the school-enterprise collaborative teaching mode based on PBL-CDIO in this paper. The pre-test sig values of professional knowledge and basic work ability of the experimental and control groups are greater than 0.05, and there is no significant difference between the two groups. The posttest sig values of the dimensions of professional knowledge in the experimental group increased by 10.20, 10.46, 10.49 and 9.47 respectively, and the sig values of the dimensions of basic work competence increased by 9.89, 9.72, 8.66 and 10.10 respectively. The overall change in the level of professional knowledge and basic work competence in the control group was less than 1 point. The posttest expertise and basic work ability sig of both groups were less than 0.05. After the experiment, the expertise and basic work ability of the experimental group were much better than that of the control group. The school-enterprise cooperative teaching mode based on PBL-CDIO proposed in this paper has good teaching effect.
This paper constructs a two-party evolutionary game model based on the perspectives of sharing platforms and consumers, exploring the dynamics of platforms’ decisions to actively operate with blockchain technology and the evolution of consumer rights protection behaviors. It is discovered from analysis that certain variables exert considerable influence on the stability of the strategies of both parties. From the consumer perspective, the improvements in the performance of the blockchain technology significantly increase the consumers’ willingness to protect their rights: the consumers with initially high levels of rights protection activation intensified their actions when their rights were violated. Thus, with the effective reduction of the cost of safeguarding rights, this trend has been additionally strengthened. As for the platform side, the performance of the blockchain technology exerts positive incentives on the operation of the platforms, although the marginal impact gradually declines with the developing blockchain technology, which in return reveals that platforms need to pay attention to a range of aspects including technology maturity. Measures of dual constraints including heavy fines from government and negative impacts of passive operations help to rein in passive operation among the platforms. Significantly, higher values of fines or negative effects lead to higher tendencies of having proactive operation strategy among the platforms.
Objective, to investigate the correlation between abdominal aortic calcification and paravertebral muscle degeneration, and to explore possible common risk factors for both. Methods, all patients with lumbar spinal stenosis admitted to Hospital X for MC and CT examination from 2016 to 2024 were selected, and through screening and exclusion, a total of 352 patients with LSS were included in the study, which consisted of 202 males and 150 females aged 40-80 years, with a mean of 63.24 years. The degree of paraspinal muscle degeneration in lumbar MRI, the degree of abdominal aortic calcification in lumbar CT scanning, as well as the patient’s age, duration of LSS, glomerular filtration rate and other indicators were counted, and the distribution characteristics of abdominal aortic calcification and its correlation with paraspinal muscle degeneration were analyzed by the method of multiple regression. Results, of the 352 patients with LSS who were included to meet the criteria, the calcification group (151, 42.90%) and the non-calcification group (201, 57.10%). Mild, moderate and severe paravertebral muscle degeneration accounted for 56.53%, 28.69% and 14.77%, respectively. The AACS in patients with mild PD degeneration stage, moderate PD degeneration stage and severe PD degeneration, all showed a gradual increasing trend with age (P<0.001). Regression results showed that age, paravertebral muscle degeneration and eGFR were risk factors for AAC in patients with LSS. Conclusion, there was a significant correlation between abdominal aortic atherosclerotic calcification and paravertebral muscle degeneration (P<0.001), and the degree of PD degeneration can be used as an effective indicator for early warning of the occurrence of AAC in patients with LSS.
In today’s society, ancient cities, as important components of historical and cultural heritage and urban development, are receiving increasing attention for their protection, utilization, and management. This research mainly focuses on the construction of an evaluation system for the spatial historical evolution of ancient city streets and the corresponding management strategies. Through a comprehensive evaluation of the spatial issues and characteristics of the ancient city streets, a multi-dimensional evaluation system for the historical evolution of the ancient city space with a total of 13 indicator factors, including historicity, is constructed. Taking Suzhou Ancient City as an example for empirical analysis, five typical types of ancient city streets are identified. Finally, corresponding update strategies are proposed for different types, especially the utilization of biomaterials and the design of plant landscapes, providing more innovative and sustainable management suggestions for the revitalization planning of the ancient city.
In this paper, the structures of three phosphorus-containing organosilicon compounds, including N,N-di-methylenephosphoric acid n-propylamine (DPPA), N,N-di-methylenephosphoric acid aminopropyldimethylsilanol (DPDS), and N, N-di-methylenephosphoric acid aminopropyldimethylsilylene glycol (DPMS), have been designed by using a molecular dynamics simulation method. And the preparation of three phosphorus-containing organosilicon compounds was accomplished experimentally by using raw materials such as bisphenol A-type epoxy organosilicon, n-propylamine and phosphite. The structures of the above several substances were proved by means of characterization such as Fourier infrared spectroscopy, hydrogen NMR , and epoxy value. Molecular dynamics simulation analysis revealed that the bond lengths of N atoms to Si atoms, N atoms to O atoms, and N atoms to were 3.03 Å, 3.05 Å, and 2.85 Å, respectively. Si did not participate in the addition reaction, but the intermolecular interactions caused a change in the chemical environment of Si, which reduced intermolecular distances and made it easier for the phosphorus groups to aggregate. This study is very important for the development of new preparation strategies of phosphorus-containing organosilicon and the promotion of phosphorus-containing organosilicon industry.
Social network structural characteristics of top management (TMT) are important variables that affect the outcome of team functioning, and variability in network structural characteristics leads to variability in TMT performance. This paper analyzes TMT social network structure characteristics based on TMT’s social relationship network using machine learning techniques. The top management interlocking network and technological innovation (machine learning technology) are divided into dimensions respectively, and the machine learning technology is used as a mediating variable to establish a model of the mediating effect of machine learning technology between top management interlocking network and green innovation. Statistical analysis of sample data and structural characterization of TMT social relationship networks by machine learning technology are conducted, and regression equations are used to verify the research hypotheses. The test results of the mediating effect of utilized innovation and exploratory innovation covered by the machine learning technology show that the overall regression effect of the model is good ( =0.537, =0.579, F-statistical test is significant), i.e., the mediating variables, utilized innovation and exploratory innovation, positively affect the green innovation performance and are significant. Meanwhile, the heterogeneity and size of TMT’s social relationship network, as well as relationship strength and relationship quality all have a significant and positive effect on green innovation.
Industrial processes are constantly developing towards large-scale and automation, and the smooth, safe, high-quality, and efficient operation of industrial processes has become a hot spot of concern, and higher requirements have been put forward for the control of production processes. This study analyzes the high-dimensional data in the closed-loop system of industrial network based on the HOPLS-SVM algorithm with higher-order singular value decomposition method. A BP neural network model is constructed with the processed data as the input set to realize the real-time prediction of the main data in the project, and the error of the prediction model is corrected by using linear regression method, and then the project prediction control system is constructed by piggybacking on the model. The results show that the prediction performance of the model in this paper is better than that of the comparison model, and the average absolute error is only 0.0347. At the same time, it is found that the control system in the decomposition project of CHP is able to regulate and optimize the temperature and flow rate of the crude product, which ensures the balance between the product temperature and yield, and the safety of the project operation. The engineering control method designed in this study has strong adaptability and effectiveness, and can provide solutions to engineering control problems in complex industrial processes.
This paper designs a military intelligent wearable device, aiming at realizing human-computer interaction and monitoring military status signs and data characteristics through this device. First of all, the overall system of the product contains temperature and humidity module, blood pressure detection module, heart rate measurement module and display module, and the extracted feature data are subjected to data intelligence preprocessing. Then the pre-processed feature data is functionally compressed and an artificial intelligence feature classification model is constructed, through which the compressed feature data is analyzed and displayed. Finally, the interactive performance of the wearable device is completed through data intelligent processing and device relevance calculation. After application analysis, it is found that the actual monitoring error is below 0.1 under different fatigue levels, and the specificity and positive prediction value of the wearable system can reach up to 100%. The highest accuracy of monitoring the physical state of military personnel is 99.31%, in addition to monitoring the heart rate in sedentary state and exercise state, with an average error value of 1.24 and 1.29. Therefore, the smart wearable device designed in this paper can well realize human-computer interaction, and the performance of product design is superior.
1970-2025 CP (Manitoba, Canada) unless otherwise stated.