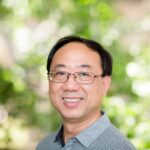
This paper first introduces the regional power marketing management platform, after which the 3 major functional modules of this power marketing management platform are designed. Then MobileFaceNet is used as the basic network for face recognition feature extraction in the context of deep learning, and the SE module is used to optimize the network performance and network expressiveness. Afterwards, the Taylor expansion of the negative log-likelihood function is used as an optimization criterion to optimize the face detection model (MTCNN) and the face recognition model (SE-MobileFaceNet). Finally, the running effect and performance of SE-MobileFaceNet model are measured. The main conclusions are as follows: in 1:1 mode, the accuracy of SE-MobileFaceNet model for the three datasets DRDS, DE and DPDS is 95.99%, 96.98% and 98.83%, respectively. In addition, the SE-MobileFaceNet model can avoid excessive redundant calculations, so that its recognition rate reaches 95%.The accuracy of the SE-MobileFaceNet model for monitoring and recognizing the information of the management platform ranges from 97.43% to 100%, and it has a good operating effect in the identification of the regional electric power marketing management information platform, and the overall satisfaction rate of the testers for the model is also >85%. The overall satisfaction of the testers to the model is also >85%. Obviously, the SE-MobileFaceNet model proposed in this paper has a very broad application in regional power marketing management information platform identity recognition.
The emergence of digital humanities echoes the paradigm transformation of literary research. In this project, natural language processing technology is applied to the analysis of ancient Chinese literature, and the attention mechanism is embedded in iterative null convolutional network to build a named entity recognition model based on ancient literature. Then we integrate MacBERT pre-training model, combine the dual-channel structure of aspectual word features and semantic features, design hierarchical attention mechanism, and complete the construction of aspectual-level sentiment analysis model. Through experiments to analyze the model performance and conduct the analysis of ancient literary works, the results can be obtained that the two models show better named entity recognition and sentiment analysis effects, and the values of each evaluation index are above 83%. Craftsmen (44.7%) and merchants (22.4%) appear more frequently in the sample Ming Dynasty fictional characters, revealing the social and cultural background of the development of the commercial economy and the emergence of the civic class at that time. Positive emotions accounted for 67.9% of the poetry samples of the Tang Dynasty, and the war of the country’s reward (0.334) and send-off feelings (0.226) co-occurred most frequently with positive emotions, reflecting the social and cultural background of the Tang Dynasty, which was characterized by prosperity and affluence, and the emergence of the literati to build up their careers.
The development of urbanization is rapidly changing, and various undertakings are flourishing, while the sports industry, as an important segment of urban regional economic development, plays an inestimable role in the development of the entire city construction. The study takes the sports industry and economic development of 27 provincial capital cities in China from 2018 to 2022 as the research object, establishes the evaluation indexes for the high-quality development of the sports industry based on the principle of index construction, and establishes the weights of the indexes. Taking Harbin as a case study, the effect between urban sports industry and economic growth is analyzed with the help of impulse response analysis, Granger causality test, and variance decomposition of VAR model. The results show that the development of urban sports industry and economic growth can promote each other, with a long-term cointegration relationship, and the positive effects between the two are slowly reduced over time when they are impacted in the long term. Granger test shows. It indicates that there is a unidirectional causal relationship between urban sports industry and economic growth.
Flexibility control and vision of robots are important acquisition and feedback links in robot control, and the study of multi-sensor data fusion is becoming more and more important as the complexity of robot tasks increases. This paper describes the robot kinematics and inverse kinematics process by studying the knowledge of D-H model theory and parameter definitions in the machine kinematics model, reveals the changing relationship between the robot joint control and end pose, and establishes a kinematics-based vision servo control model. On this basis, the coupling error compensation algorithm is used to combine the visual position control quantity as well as the force sensing position correction quantity to form the final visual and force sensing supple control strategy. Meanwhile, for the lack of adaptability of classical impedance force control on unknown constraint environments, a two-fuzzy adaptive sliding mode controller is designed according to the Lyapunov stability theorem to drive the robot end in order to achieve the actual position tracking expectation. The results of simulation experiments and motion contour tracking experiments show that the control algorithm proposed in this paper has better control accuracy and is more robust to noise and uncertainty, and the controller is also able to reduce the effect of torque saturation on the robot system.
Garden is an important support for regional economic development, but also an important support for regional ecological environmental protection, the rational allocation of water resources in the garden is one of the effective ways to solve the problem of water shortage. This paper takes the Internet of things, digital twin as the technical basis, uses the multi-objective optimisation algorithm to construct the water resource management model of the garden area, and uses the artificial fish swarm algorithm to solve the model. By constructing a digital twin irrigation district water resources scheduling management platform, the water resources elements of the garden area are comprehensively monitored and sensed, and the intelligent simulation of the water resources allocation management process and decision-making scheme evaluation and optimisation are achieved, so as to enhance the intelligent and refined management level of water resources scheduling of the garden area, and comprehensively realise the saving and intensive use of water resources. Taking X garden area as a research case, the water resources management model finally derives the optimal water resources allocation scheme under 50%, 75% and 90% in each planning year, which provides support for the efficient use of water resources in X garden area.
This paper evaluates the quality of university English teaching based on the hierarchical analysis algorithm (AHP) and fuzzy comprehensive evaluation algorithm (FCEA) in order to grasp the teaching situation more objectively. Based on the principle of evaluation index system construction, the evaluation indexes of university English teaching quality are determined. Using hierarchical analysis algorithm to calculate the weights of its indicators, and constructing a fuzzy comprehensive evaluation matrix based on expert ratings to finalize the assessment of university English teaching quality. Taking a university as the research subject, the English teaching quality assessment result of the university is 3.7351, and its corresponding fuzzy comprehensive evaluation A=(0.2893,0.3981,0.1359,0.1120,0.0648), which summarizes the teaching quality of university English as good according to the principle of maximum affiliation degree. In order to improve the teaching of college English in this university, corresponding teaching strategies of college English are proposed.
Deep learning, as a multilayer neural network structure for deep learning of data features, can describe the nonlinear mapping relationship for the assessment of college civic education. Aiming at the current education quality assessment model based on deep learning, this paper proposes an optimized convolutional neural network (HOA-CNN) based on Hummingbird Optimization Algorithm to assess the quality of Civic and Political Education in colleges and universities. According to the correlation coefficient between the objective assessment results and the subjective assessment results, the objective assessment results of the quality of Civic and Political Education in colleges and universities are obtained. The test results show that the linear correlation coefficient and the rank correlation coefficient between the assessment results of this method and the subjective assessment results are closer to 1. The goodness-of-fit of the assessment of the quality of college civic education under the model of this paper is significantly higher than that of the two control models. The simulation test results show that the assessment results of the university civic education quality assessment model constructed by the optimized convolutional neural network based on the hummingbird optimization algorithm are more accurate.
When a steam turbine blade has cracks, fractures, or other flaws, the steam turbine’s operating circumstances will change the vibration characteristics of the blades, complicating the problem identification process. The important defect features are difficult to automatically and effectively extract from the recorded vibration signals. In this study, the input signal characteristics for a particular operating situation are used as labels to reconstruct a trained autoencoder utilizing a reverse error. The supervised autoencoder receives the fault features for various speed circumstances, which it then protectively maps to a series of reference condition features. The goal is to eliminate the disruption brought on by variations in fault feature values brought on by alterations in operating circumstances. The experimental findings demonstrate that this approach can more effectively convert feature sequences under various working situations and address the issue of fault feature distortion brought on by changes in working conditions. In addition, comparison of clustering visualization and accuracy of classification methods on data before and after commutation demonstrates that the proposed supervised autoencoder model can extract accurate classifiable features for fault classification.
The research combines PBL teaching method, CDIO theory and school-enterprise collaborative education mechanism to construct a school-enterprise collaborative teaching model based on PBL-CDIO. And then, the empirical research of PBL-CDIO school-enterprise collaborative teaching mode is realized through the teaching experiment method. The independent sample t-test is used to test the changes in the professional knowledge level and basic working ability of the experimental group and the control group before and after the experiment, and to judge the teaching effect of the school-enterprise collaborative teaching mode based on PBL-CDIO in this paper. The pre-test sig values of professional knowledge and basic work ability of the experimental and control groups are greater than 0.05, and there is no significant difference between the two groups. The posttest sig values of the dimensions of professional knowledge in the experimental group increased by 10.20, 10.46, 10.49 and 9.47 respectively, and the sig values of the dimensions of basic work competence increased by 9.89, 9.72, 8.66 and 10.10 respectively. The overall change in the level of professional knowledge and basic work competence in the control group was less than 1 point. The posttest expertise and basic work ability sig of both groups were less than 0.05. After the experiment, the expertise and basic work ability of the experimental group were much better than that of the control group. The school-enterprise cooperative teaching mode based on PBL-CDIO proposed in this paper has good teaching effect.
This paper constructs a two-party evolutionary game model based on the perspectives of sharing platforms and consumers, exploring the dynamics of platforms’ decisions to actively operate with blockchain technology and the evolution of consumer rights protection behaviors. It is discovered from analysis that certain variables exert considerable influence on the stability of the strategies of both parties. From the consumer perspective, the improvements in the performance of the blockchain technology significantly increase the consumers’ willingness to protect their rights: the consumers with initially high levels of rights protection activation intensified their actions when their rights were violated. Thus, with the effective reduction of the cost of safeguarding rights, this trend has been additionally strengthened. As for the platform side, the performance of the blockchain technology exerts positive incentives on the operation of the platforms, although the marginal impact gradually declines with the developing blockchain technology, which in return reveals that platforms need to pay attention to a range of aspects including technology maturity. Measures of dual constraints including heavy fines from government and negative impacts of passive operations help to rein in passive operation among the platforms. Significantly, higher values of fines or negative effects lead to higher tendencies of having proactive operation strategy among the platforms.